Financial Technology: Ripe For Disruption
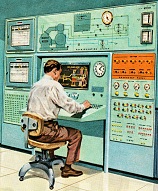
Editor’s Note: This is part of a series highlighting the role technology may play in bringing about the Future of Finance.
The use of technology in finance is not a subject that investment professionals frequently think about — unless it is not working. But being an investor in early-stage start-up companies, I’m particularly excited about opportunities for new technology companies in financial services. In fact, looking back over the past 20–25 years, I would argue that there has not been a better time to invest in financial technology companies than right now.
That may seem overly bullish, but there are some capabilities and trends today that back up my stance. First, if you are working in financial services today, I would bet you large sums of money that many of your tools are old. Furthermore, I would bet that the tools that are not old are mostly home-grown and used for proprietary parts of your business. For those in some parts of the market, building proprietary software and algorithms is a key competitive advantage; I’m not talking specifically about those types of software tools. Those programs won’t be replaced easily by a standard tool (although I wouldn’t be surprised if someone makes creating, testing, and running algorithms very easy).
What I see is an industry that has not had a lot of innovation in its systems in years. Some of the new cloud-based software-as-a-service (SaaS) capabilities will enable existing technology to make quantum leaps. I see this possibility in all areas of finance, but in order to keep from getting too broad, I will focus on asset management and investment decision making.
Most investment professionals would say that their real-time decision-making tools, especially those related to trading of publicly listed securities, are quite good and have continually advanced. Quantitative trading and proprietary algorithms have been leading areas of innovation in financial technology, and since 1999, we have seen high-frequency trading become commonplace, particularly after 2005. Today, high-frequency trading accounts for more than half of all orders in the United States. Ultra-low latency direct market access (ULLDMA) systems can boast round-trip execution under 10 milliseconds. The systems that carry out these trades and support the proprietary trading algorithms are some of the pioneering technology advances that will be the next wave of innovation in financial technology.
However, beyond the trading and proprietary quant systems, the tools and technology tend to fall off a cliff. Analysts who are not quant jocks or programmers don’t have the same capabilities as those who are and often have to rely on more traditional resources for a portion of their analysis, or they use Excel to the best of its ability. Excel is a great tool — I use it a lot — but it isn’t nearly as powerful as many other tools. MATLAB and other leading mathematical modeling tools, however, don’t extend easily to share data or to receive large amounts of data from disparate sources.
Analysts today find correlations between seemingly non-related data, and as “big data” become more available, the need for more sophisticated tools to manage and analyze these data grows. Companies such as Palantir and its product Metropolis are moving in this direction and are already solving some difficult problems, but Palantir will be the first to tell you that there is far more to do. Metropolis can enable very complex analysis without requiring programming work and allows for seamless sharing; this product is empowering analysts in ways that have not been done previously, and it is able to work side-by-side with proprietary quant systems. This is one example where I see some impressive innovation that is making an impact. But I’m looking for more.
Huge amounts of valuable data are available, but the standard systems in place today were mostly built before these massive amounts of data were being collected and before the widespread use of the application programming interface (API). Standard systems simply can’t handle it. Many of the standard systems were built with technology from the 1980s and 1990s, when client servers, Windows, relational databases, middleware, and ETL (extract, transform, and load) processes were the name of the game. These systems have, by and large, evolved by making the front end nicer — by using a web-based front end or by making some functionality available on mobile devices — but core systems haven’t evolved very much because it is extremely risky and difficult to take a system that is being used and change its underlying architecture. It would be like trying to keep the outside of the house intact while redoing the foundation and all the supporting structures. Even systems from the 2000s, which are primarily web based, tend to fall behind the new generation of big data systems.
Think about the ability to pull in real-time data from social media, transactional retail (online and offline) data, mobile patterns, supply chain data, and many other types of data from different industries, regions, and sources. Integrating that information with market data to identify patterns very early can be extremely powerful. Non-market data are becoming more available globally as well, and some of these data will undoubtedly produce additional signals that can be used for proprietary trading and investment selection. I know some teams are already moving in this direction.
The next generation of investment analysis tools will necessarily be built around big data and will enable the examination of massive amounts of data, including data that are often unstructured and may not even be assumed to be relevant until the multivariate analysis is done. These systems will enable us to move beyond the somewhat standard models used today, in which the inputs are adjusted based on quarterly numbers and other inputs are used to generate buy/sell recommendations and price targets. With more data and more timely data, the potential to be ahead of the curve and to have stronger signals will continue to improve selection capabilities. As these systems get further integrated into the advanced quant systems, we will be able to better leverage the skills and capabilities of analysts, quant teams, and entire organizations to generate value.
As big data infiltrate deeper into the world of investment selection and trading, I believe we are going to see giant leaps in capabilities and witness new frontiers developing.
If you liked this post, don’t forget to subscribe to Inside Investing via Email or RSS.
Please note that the content of this site should not be construed as investment advice, nor do the opinions expressed necessarily reflect the views of CFA Institute.
Photo credit: iStockphoto/CSA_Images